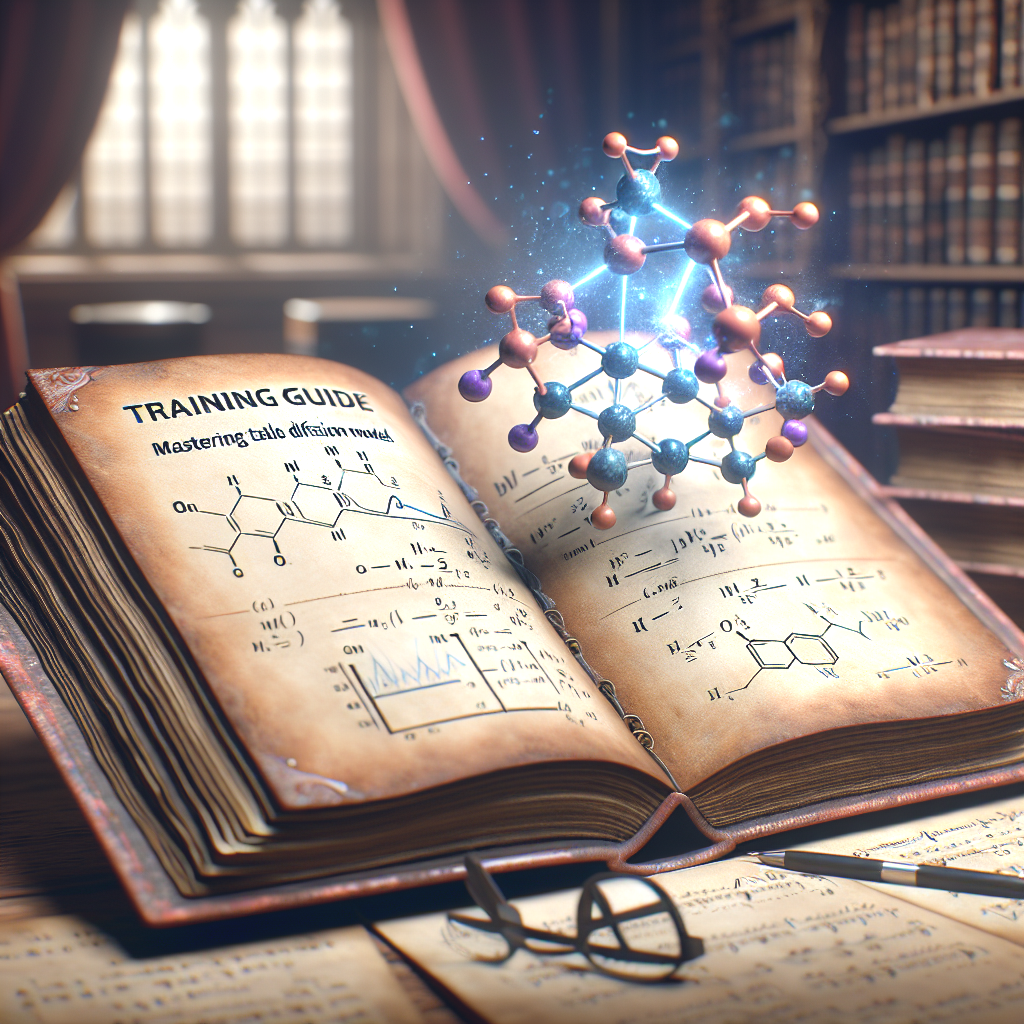
Mastering Stable Diffusion Models: A Training Guide
- Artificial Intelligence in Content Creation: A Focus on Stable Diffusion Models
- An Overview of Stable Diffusion
- Training Stable Diffusion
- Data Preparation for Stable Diffusion
- Designing the Stable Diffusion Model
- Training Stable Diffusion
- Important Considerations
Artificial Intelligence in Content Creation: A Focus on Stable Diffusion Models
The current technological era has seen an incredible surge in the employment of artificial intelligence (AI) tools for content creation in 2023. In a market that's saturated with diverse AI capabilities, it's often tough to keep up. This article will delve into one of these tools, stable diffusion, and guide you on how to effectively train it.
Since the renowned rise of OpenAI's ChatGPT, AI tools have become indispensable components of modern workflows. They are now highly influential in various fields, with a critical role in AI-driven image generation. Stable diffusion stands out among these tools, given its fascinating capability of generating impressive outcomes.
An Overview of Stable Diffusion
The growing interest in AI tools has led to the creation of several generative applications. Among these, Stable Diffusion is one of the more popular ones. Stable diffusion models employ generative AI algorithms that leverage machine learning (ML) and historical data to predict results or events.
Pivotal to the function of these models is the diffusion process. The diffusion process adds noise to an image then gradually diminishes it, leaving behind a final, refined image. The ML technique fundamental to the diffusion model has been observed to yield better results than deep learning models (DL).
Apart from being intelligent and powerful, these algorithms can handle complex prompts and instructions, allowing users to describe their needs with precision. Stable diffusion represents the latest evolution in AI image generation, using the ML model to generate high-quality images from specified inputs.
Training Stable Diffusion
Understanding the training process of the image-generating algorithm is crucial for anyone seeking to use it. The primary method of training stable diffusion models is adversarial training, which involves two models trained against each other - the generator model and the discriminator model.
The generator model works to create the most realistic images possible, while the discriminator model tries to identify which images are real and which are AI-generated.
Data Preparation for Stable Diffusion
Before the training of a stable diffusion model begins, the necessary data must be prepared. This process consists of several steps, starting with data collection. This refers to the gathering of relevant and up-to-date data for the desired outcome.
Next is the data-cleaning process, which involves assessing and removing outliers, missing data, and other inconsistencies for improved accuracy. Then comes the preprocessing phase, with tasks like normalization, standardization, and dimensionality reduction to boost model performance.
Designing the Stable Diffusion Model
Once the data has been prepared, the next step is designing the stable diffusion model. At this stage, the algorithm, parameters, or architecture to be used for the model is chosen. Popular algorithms used in stable diffusion include deep convolutional neural networks (DCNN), generative adversarial networks (GAN), and variational autoencoders (VAE).
Training Stable Diffusion
To train the model, tools and platforms such as Google Colab, Jupyter Notebooks, and TensorFlow can be utilised. The interactivity of the environment in which the training is performed is crucial.
Important Considerations
In training your model, keep in mind a few critical factors. The quality of your data determines the effectiveness of your model, so it's crucial to use high-quality data. Different hyperparameters will influence how the model uses the data in its learning. Finally, ensure a robust training setup and monitor the progress of your model regularly.
How do you like the article?
Join the discussion on
You may also like