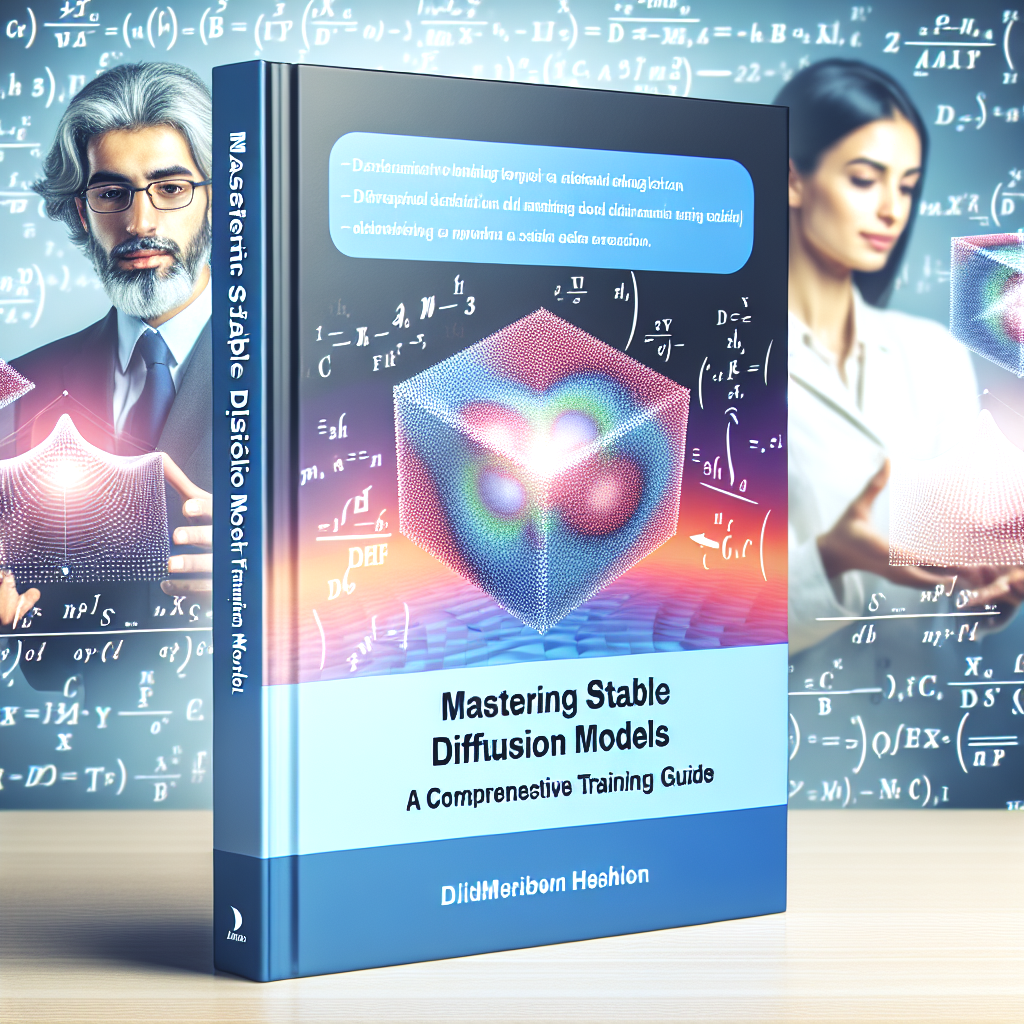
Mastering Stable Diffusion Models: A Comprehensive Training Guide
- Exploring the Mystery: Training Stable Diffusion Models in Detail
- Prologue: Gaining Insight into Stable Diffusion Models
- Blueprint: Training Stable Diffusion Models
- 1. Preparing the Environment: Google Colab as Your Training Field
- 2. Assembling the Tools: Images as the Training Base
- Training Concerto: Steps in the Process
- 1. Beginning the Journey: Training the Primary Model
- 2. Refining the Performance: Fine-Tuning for Accuracy
- 3. The Element of Surprise: Incorporating Random Noise
- Perfecting the Dance: Best Practices for Training
- 1. Understanding the Long Haul: Time Investment
- 2. The Importance of Model Design: Carving Your Unique Path
- 3. Validation: Guaranteeing Quality during the Creative Process
- Finale: Generating Images with Trained Stable Diffusion Models
- Epilogue: Your Path as a Stable Diffusion Maestro
Exploring the Mystery: Training Stable Diffusion Models in Detail
Stable Diffusion Models have emerged as a revolutionary aspect of machine learning, noted for their capacity to generate high-resolution imagery. In this detailed exploration, we will dissect the process for training Stable Diffusion Models, providing the knowledge needed to effectively utilize their image generation capabilities.
Prologue: Gaining Insight into Stable Diffusion Models
Before delving into the details of training, it's essential to understand the core of Stable Diffusion Models. At the intersection of deep learning and image generation, these models function through a diffusion process that progressively enhances images. This results in distinct images that embody a perfect blend of realness and creativity.
Blueprint: Training Stable Diffusion Models
1. Preparing the Environment: Google Colab as Your Training Field
Google Colab emerges as a powerful platform for training Stable Diffusion Models. Its cloud-based functionality and offering of free GPU resources make it a suitable setting for exploring deep learning complexities without the pressure of hardware limitations.
2. Assembling the Tools: Images as the Training Base
The crux of training Stable Diffusion Models resides in the selection of training images. These function as the raw input that the model fine-tunes during training. In addition, a varied and representative dataset allows the model to learn the various aspects of different visual elements.
Training Concerto: Steps in the Process
1. Beginning the Journey: Training the Primary Model
The training initiates by introducing the base Stable Diffusion Model to the chosen training images and allowing it to embark on the diffusion process. The objective at this stage is to lay the groundwork for ensuing refinement, rather than achieving perfection.
2. Refining the Performance: Fine-Tuning for Accuracy
Fine-tuning forms the essential ingredient in the Stable Diffusion Model training symphony. Once the base model has navigated through the initial training phase, fine-tuning steps in to sharpen the model's comprehension. This step entails a meticulous process of modifying parameters and optimizing the model for the specific traits of your selected dataset.
3. The Element of Surprise: Incorporating Random Noise
An integral part of training Stable Diffusion Models is the integration of random noise. This element accelerates the diffusion process, introducing an element of unpredictability and ingenuity. The model learns to enhance and navigate images while embracing the inherent randomness of the creative process.
Perfecting the Dance: Best Practices for Training
1. Understanding the Long Haul: Time Investment
Keep in mind, this process is a marathon, not a sprint. The complexities of the diffusion process and fine-tuning require time and computational resources. Show patience and allow the model the time it needs to evolve into a creative dynamo.
2. The Importance of Model Design: Carving Your Unique Path
The design of your Stable Diffusion Model is comparable to a tailor-made recipe. Experiment with various neural network architectures, adjusting and perfecting until you discover the perfect combination for your specific application. Model design is the craftsmanship that turns training into a fine art.
3. Validation: Guaranteeing Quality during the Creative Process
Validation is the safeguard that ensures the quality of your trained model. Dedicate a portion of your dataset for validation, allowing you to measure the model's performance on images it hasn't seen during training. Crucially, this step prevents overfitting and assures the generalization of your model.
Finale: Generating Images with Trained Stable Diffusion Models
As your Stable Diffusion Model concludes its training journey, you have arrived at the gateway where machine learning and creativity intersect. With a well-tuned model, you are now ready to generate images that echo the artistic spirit of your training dataset.
Epilogue: Your Path as a Stable Diffusion Maestro
Training Stable Diffusion Models is a dynamic journey that necessitates a blend of technical proficiency and creative intuition. Absorb the learning process, try different strategies, and delight in your model's development into a self-sufficient artist. In conclusion, the field of Stable Diffusion Models presents unparalleled prospects at the crossroads of machine learning and artistic expression. With this guide in hand, you are ready to embark on your journey to mastering the training of Stable Diffusion Models and unlocking the creative potential in image generation.
How do you like the article?
Join the discussion on
You may also like