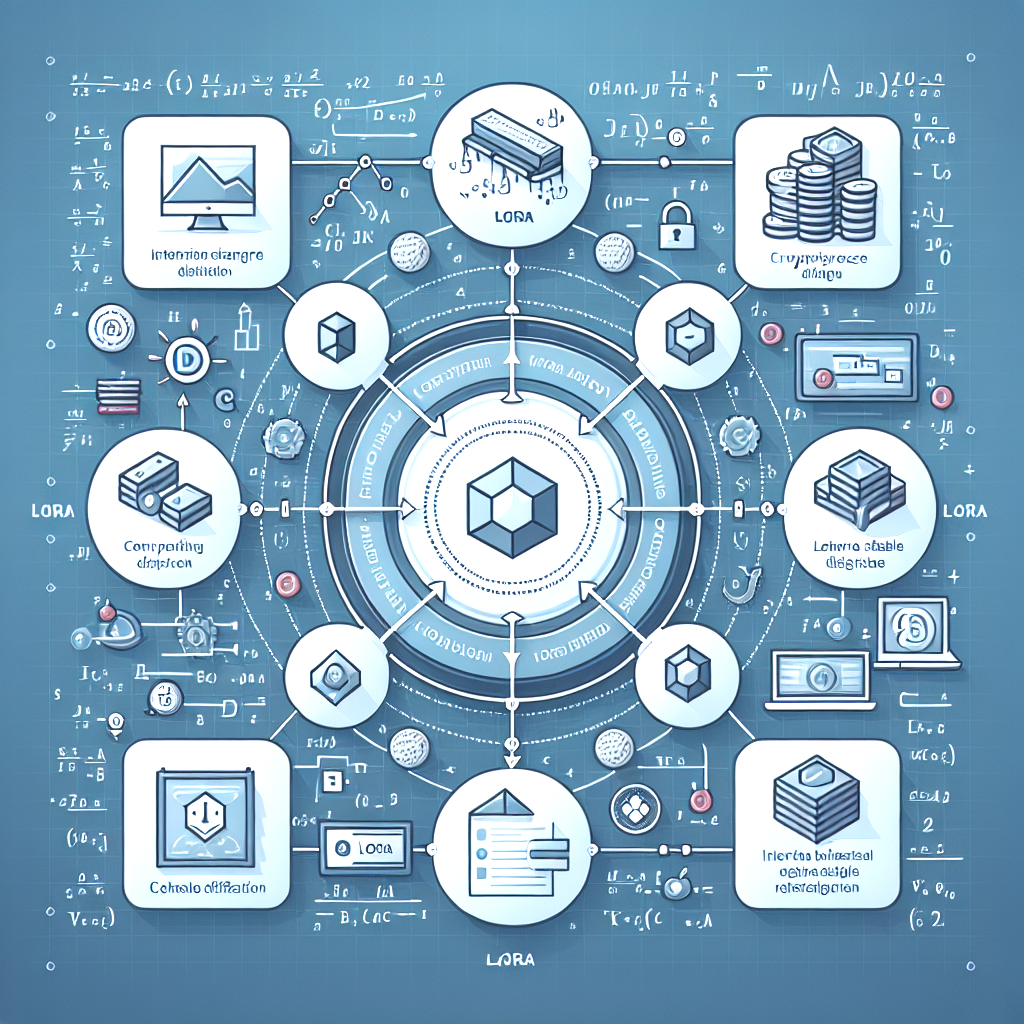
Guide: Adding Lora with Weight Stable Diffusion in Crypto
- The Comprehensive Guide: Incorporating Lora with Weight Stable Diffusion
- Diving Into the Basics: Understanding Lora and Stable Diffusion
- Demystifying Multiple Lora
- Exploring Weight Stable Diffusion
- Mastering the Integration Process
- Initiating the Fusion: Refining Stable Diffusion Models with Lora
- The Significance of Batch Size and Cross-Attention Layers
- Refinement for Precision: Setting Checkpoints
- Overcoming Text Error and Text Encoder Challenges
- Raising the Bar: Leveraging Lora Weights
- Comparing the Original and Enhanced Models
- Revolutionizing the Future: A Fine-Tuned Model
- The Power of Lora Trained Stable Diffusion
- Implications and Applications
- Conclusion: Spearheading the Next Innovation Wave
The Comprehensive Guide: Incorporating Lora with Weight Stable Diffusion
In the dynamic world of machine learning, embracing novel techniques is essential. A groundbreaking innovation grabbing significant attention is the integration of multiple Lora with Weight Stable Diffusion. This guide aims to delve into the complexities of this collaboration, providing a stepwise method to boost your model's performance.
Diving Into the Basics: Understanding Lora and Stable Diffusion
Demystifying Multiple Lora
With an increasing prominence in diverse machine learning applications, Lora possesses a unique array of capabilities. The integration of multiple Lora facilitates a more sophisticated approach to interpreting intricate patterns and generating varied outputs. In this journey, it is vital to understand the synergy between Lora and the Stable Diffusion model.
Exploring Weight Stable Diffusion
Known for its stability in generating high-quality images, Stable Diffusion forms the basis of our exploration. Through the incorporation of weights into the Stable Diffusion model, we strive to refine its performance, thereby achieving a nuanced balance that augments precision and versatility.
Mastering the Integration Process
Initiating the Fusion: Refining Stable Diffusion Models with Lora
The integration process kickstarts with the refinement of Stable Diffusion models utilizing multiple Lora. This involves tweaking the weights, an integral step to attain a harmonious partnership between the models. When getting into the codebase, ensure to work with Stable Diffusion v1.5 or later to leverage the model's advancements.
The Significance of Batch Size and Cross-Attention Layers
Optimizing the batch size becomes critical when dealing with the fusion of Lora with Stable Diffusion. Modifying this aspect contributes to the model's efficiency and performance. Moreover, the incorporation of cross-attention layers boosts the model's ability to focus on relevant features, promoting more accurate and context-aware image generation.
Refinement for Precision: Setting Checkpoints
It is advisable to create regular checkpoints for your models throughout the integration process. This ensures a safety net in case of unforeseen errors or changes. This step is highly significant, particularly when handling experimental enhancements like integrating multiple Lora with Weight Stable Diffusion.
Overcoming Text Error and Text Encoder Challenges
While refining Stable Diffusion models with Lora, you may face challenges associated with text errors and text encoders. Be meticulous about these aspects, modifying parameters and configurations to rectify any inconsistencies and improve the model's understanding of text input.
Raising the Bar: Leveraging Lora Weights
The integration of Lora with Stable Diffusion demands a thorough understanding of Lora weights. These weights hold a substantial impact on the model's decision-making process and outputs. Experiment with different weight configurations to find a balance aligning with your specific requirements and objectives.
Comparing the Original and Enhanced Models
Throughout the integration process, make sure to periodically compare the outputs of the original Stable Diffusion model with the modified version, which incorporates multiple Lora. This comparative analysis offers invaluable insights into the effects of the integration, guiding further refinements for superior performance.
Revolutionizing the Future: A Fine-Tuned Model
The Power of Lora Trained Stable Diffusion
Upon successful integration, the model transforms into a potent tool with improved capacities in image generation and pattern recognition. The unification of Lora with Weight Stable Diffusion enhances the model's proficiency, making it capable of tackling diverse tasks in the machine learning arena.
Implications and Applications
The consequences of integrating multiple Lora with Weight Stable Diffusion are extensive. This refined model paves the way for inventive applications across various fields, from creative image generation to sophisticated pattern recognition. When exploring these possibilities, bear in mind the ethical implications and responsible usage of your advanced model.
Conclusion: Spearheading the Next Innovation Wave
Integrating multiple Lora with Weight Stable Diffusion denotes a major leap in the evolution of machine learning models. By understanding the fine-tuning complexities and harnessing the cooperative power of Lora, you pioneer the next innovation wave in this field.
How do you like the article?
Join the discussion on
You may also like