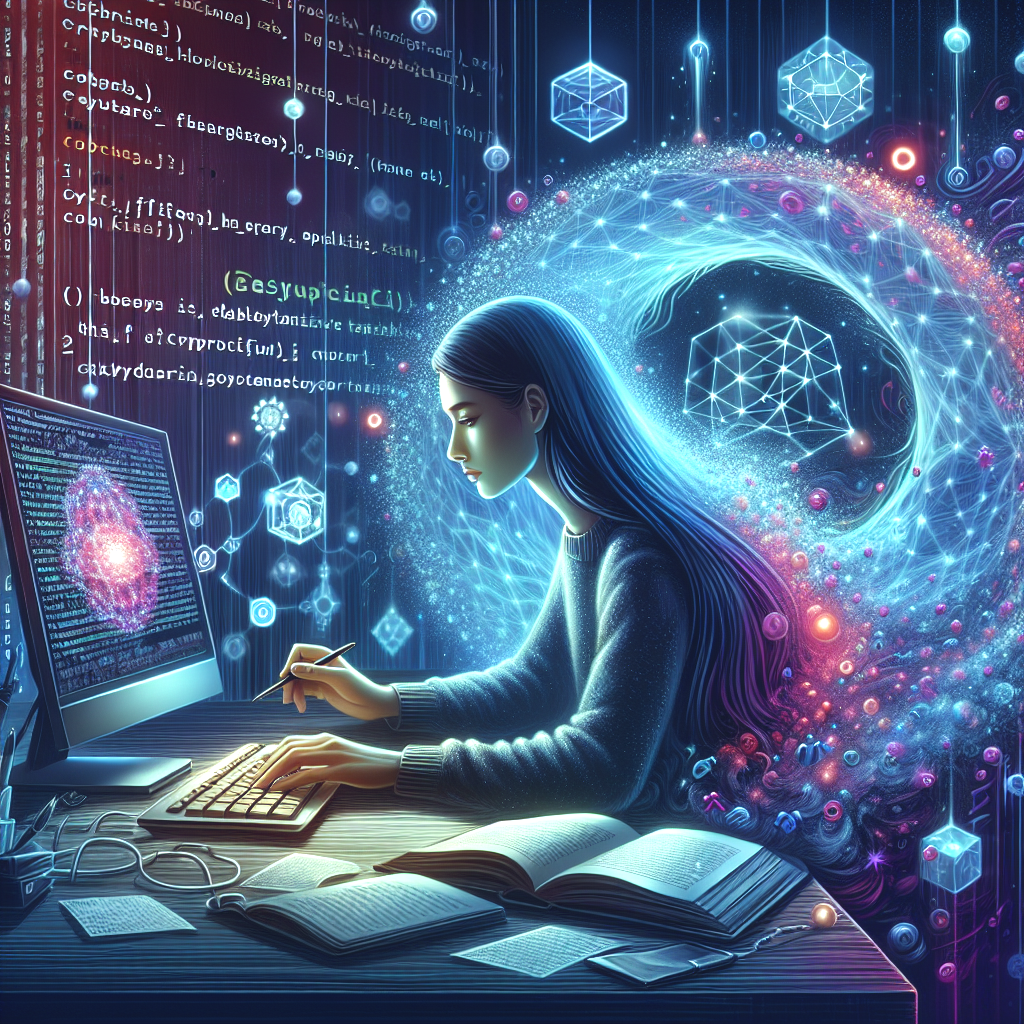
Mastering Stable Diffusion Training in Crypto
- Mastering Stable Diffusion Training: A Comprehensive Guide
- Essential Principles of Stable Diffusion Training
- Understanding the Fundamentals
- The Significance of Google Colab
- The Journey to Training Stable Diffusion
- Preparation of Training Images
- Model Input: Transforming Random Noise into Art
- Model Evaluation: The Road to Excellence
- Building a Robust Stable Diffusion Model
- A Strong Dataset and Robust Model
- Strategic Model Design
- Optimizing Efficiency in Training
- Final Stages: Bringing Your Model to Life
- Generating Final Images
- Continuous Learning and Refinement
- Conclusion: Advancing Your Stable Diffusion Skills
Mastering Stable Diffusion Training: A Comprehensive Guide
Delve into the fascinating world of Stable Diffusion where machine learning and artificial intelligence synergize to create awe-inspiring images. This exhaustive guide will simplify the complex aspects of training Stable Diffusion models, exploring everything from leveraging the power of Google Colab to understanding subtle aspects of model design.
Essential Principles of Stable Diffusion Training
Understanding the Fundamentals
Stable Diffusion students must first grasp the basics: the model creates images by progressively refining random noise. This process, steered by neural networks, results in the formation of detailed and superior final images.
The Significance of Google Colab
Google Colab becomes an integral part of your Stable Diffusion journey providing a cloud-based platform to execute neural network tasks without affluent computing resources. Its cooperative nature ensures an uninterrupted exploration and execution of your training tasks.
The Journey to Training Stable Diffusion
Preparation of Training Images
The success of a Stable Diffusion model largely depends upon the quality and diversity of training images. Assemble a dataset that encapsulates the visual richness you aim to capture. An extensive dataset covering an array of scenarios equips your model with versatility.
Model Input: Transforming Random Noise into Art
During training, the model uses random noise as its baseline. This seemingly disorderly input serves as the foundation from which your Stable Diffusion model generates its visual masterpieces. The input of random noise marks the beginning of producing images that reflect the essence of your training dataset.
Model Evaluation: The Road to Excellence
Training a Stable Diffusion model is a repeated process. Regularly evaluate the model's development to fine-tune its parameters. This evaluation includes contrasting the generated images with your dataset, assuring the model is progressing towards your desired visual output.
Building a Robust Stable Diffusion Model
A Strong Dataset and Robust Model
The strength of a large dataset is reflected in the robustness of your model. Exposure to a variety of scenarios equips the model to handle varied input patterns, creating final images that exhibit a high level of detail and realism.
Strategic Model Design
The architecture of your Stable Diffusion model plays a crucial role in its performance. Strategic design considerations include configuring the number of layers, the dimensionality of input images, and the intricate details that shape the neural network's structure. The key to creating a model that produces high-quality images lies in balancing complexity with efficiency.
Optimizing Efficiency in Training
Efficiency plays a crucial role in the training process. By employing techniques like layer normalization and strategic initialization of weights, the convergence of your Stable Diffusion model becomes smoother. These optimizations ensure precise and rapid navigation of your model during training.
Final Stages: Bringing Your Model to Life
Generating Final Images
As your model progresses through the training epochs, the beauty of the final images begins to unfold. These images express the essence of your dataset, transformed into a visual tapestry by the intricacies of neural networks. The fruit of your training efforts is reflected in images that mirror the diversity and richness of your initial dataset.
Continuous Learning and Refinement
The journey doesn't conclude with the generation of final images. Adopt a culture of constant learning and refinement. By constantly evaluating your model's performance, introducing new training images, and discovering advanced model architectures, you can expand the capabilities of your Stable Diffusion model.
Conclusion: Advancing Your Stable Diffusion Skills
In conclusion, training Stable Diffusion models is an art that blends machine learning principles with the creative sphere of image generation. From mastering Google Colab to strategically designing your model, each step contributes to the evolution of a robust and versatile image generator. With this guide, dive deeper into the world of Stable Diffusion, navigating your way towards advanced skills and innovation in the sphere of artificial intelligence and visual creativity.
How do you like the article?
Join the discussion on
You may also like