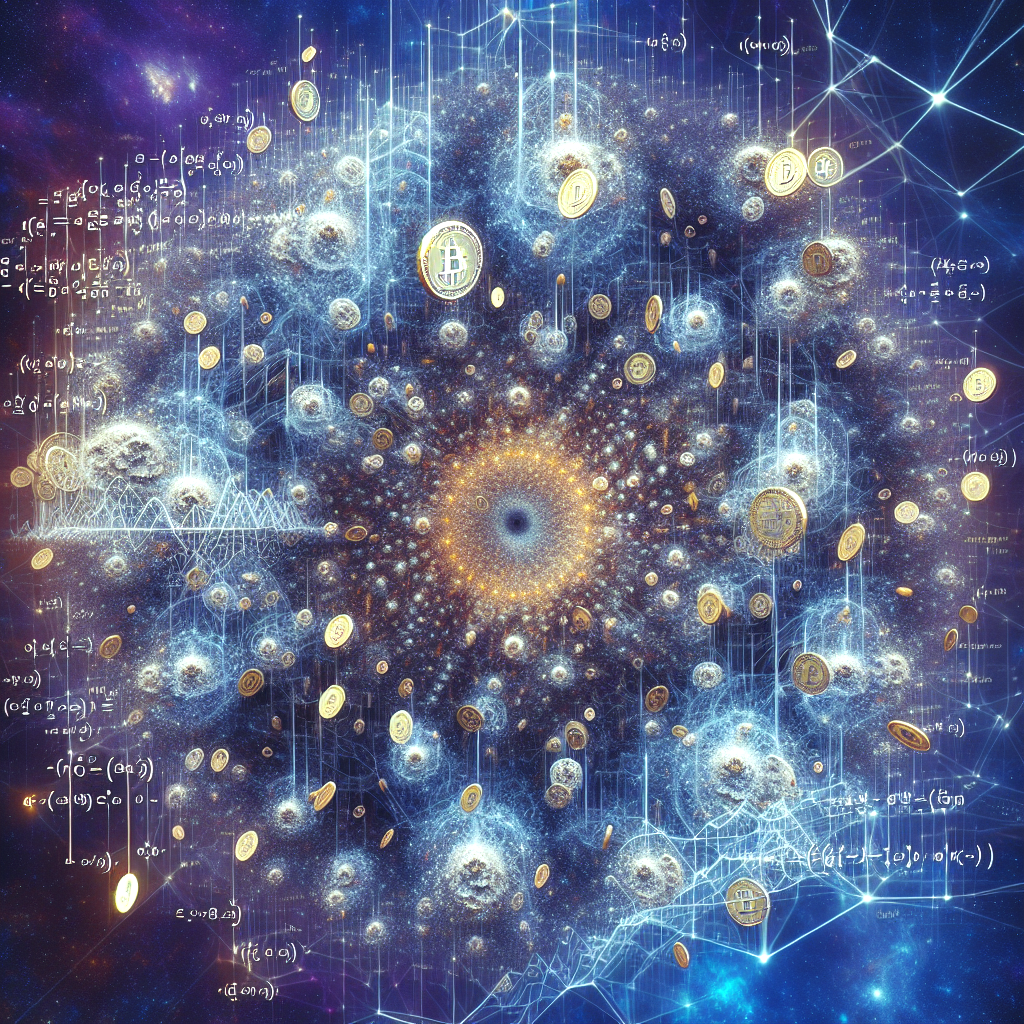
Accelerating Stable Diffusion in Cryptocurrency: A Guide
- Exploring the Impact and Optimization of Stable Diffusion in the AI Landscape
- Understanding Stable Diffusion
- Strategies to Speed Up Stable Diffusion
- Cross-Attention Optimization
- Token Merging
- Negative Guidance Minimum Sigma
Exploring the Impact and Optimization of Stable Diffusion in the AI Landscape
The advent of OpenAI's ChatGPT has undeniably pushed artificial intelligence (AI) into the mainstream spotlight this year. One of these exciting developments is Stable Diffusion, a popular image-generating program that has greatly influenced the AI space. While it comes with its own set of challenges, efforts are underway to enhance its efficiency and speed. Let's take a deeper look at Stable Diffusion and explore strategies to improve its performance.
Understanding Stable Diffusion
Stable Diffusion is an AI-based algorithm geared towards creating images. It's a part of a larger diffusion model network that has gained popularity due to its impressive results. The process of image creation begins with a blurry image that is gradually refined based on the given prompt. Despite its remarkable outcomes, the process can be quite time-consuming.
Strategies to Speed Up Stable Diffusion
While there has been considerable interest in speeding up Stable Diffusion, there isn't a simple solution. Yet, there are three main strategies that can be employed to make the output of the algorithm quicker, thereby reducing the time it consumes to create an image.
Cross-Attention Optimization
The first approach to accelerate Stable Diffusion is through cross-attention optimization. It is a successful method of speeding up the process as it is designed to enhance the cross-attention calculation of the algorithm and decrease memory usage. The best way to optimize cross-attention largely depends on the software you are using in conjunction with Stable Diffusion. For instance, when using AUTOMATIC1111 Stable Diffusion GUI, one would access the settings page, select the Optimization tab, and navigate to the Cross Attention Optimization feature.
Besides, several techniques are available for cross-attention optimization, including Doggettx, xFormers, Sub-quadratic attention, among others, that focus on speeding up the overall process.
Token Merging
Another method to boost Stable Diffusion's speed is through a technique known as Token Merging. It involves reducing the number of tokens processed by the algorithm, thereby increasing its speed. This technique identifies tokens that may be redundant or unnecessary, thus expediting the output.
Using AUTOMATIC1111, for example, you can access its built-in token merging support via the Settings page and adjust the Token Merging ratio under the Optimizations tab. Once you've set your desired amount, simply select Apply Settings.
Negative Guidance Minimum Sigma
The third strategy to speed up Stable Diffusion involves negative guidance sigma. This process turns off the negative prompt under certain conditions, which may seem unimportant but can significantly affect the outcome.
To implement this, navigate to the settings page of the program, locate the optimization tab, and set the value of the negative guidance minimum sigma. After achieving the optimal value, select Apply Settings.
While there are other methods to optimize Stable Diffusion speed like PyTorch optimization or DeepSpeed inference, these three techniques provide a robust starting point to enhance the algorithm's performance and significantly decrease its output time.
How do you like the article?
Join the discussion on
You may also like